Data-Centric AI: The advantages for engineers in various sectors through the shift from Model-Centric to Data-Centric AI
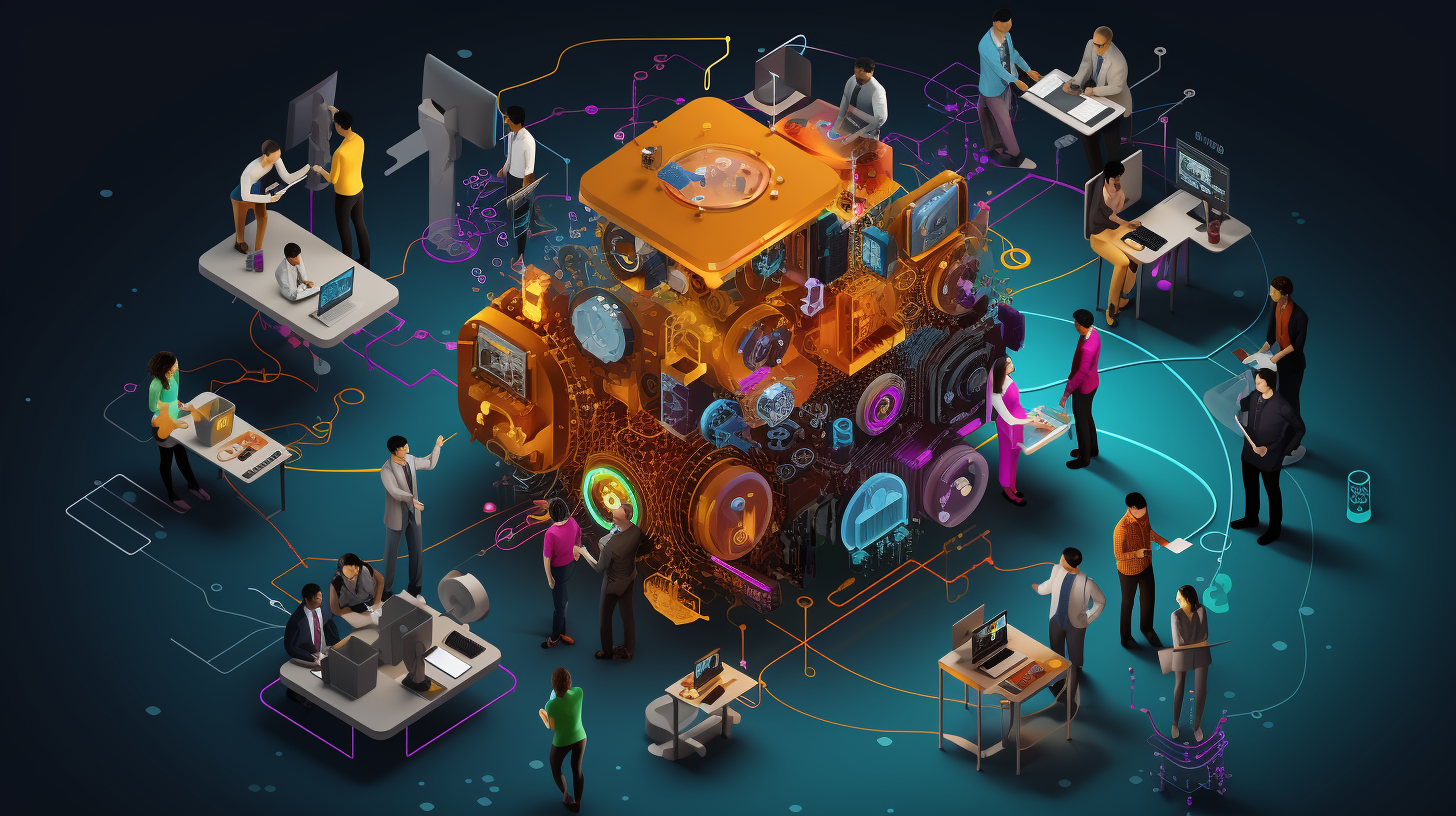
To enhance accuracy in diverse applications, many engineers have been embracing data-centric AI which differs from the traditional model-centric approach. What seems to be a prompt for most engineers to reassess their priorities and workflows is the abundance of available data and recognition of the advantages offered by reliable data. By acknowledging that a model's performance heavily relies on the quality of the training data, this emphasis on data has empowered engineers to enhance model accuracy without engaging in the repetitive process of constantly adjusting parameters.
Enhancing data quality and model precision in data-centric AI enables the exploration of new engineering applications, including 5G communications, LiDAR, medical device imaging and state of charge estimations, all of which present fresh opportunities in the field.
While careful data examination has always proven critical to successful modeling, the modern challenge lies in determining how data-centric AI should advance to solve specific application problems, and what techniques and tools are available to do so. Data-centric AI gives engineers access to new capabilities both in terms of the answers that can be found and the issues that can be addressed.
Data-Centric AI: Optimal Approaches
To ensure precise outcomes, engineers are placing greater emphasis on enhancing the quality of data utilized in models. However, while data-centric AI consistently enhances model performance, it is crucial to acknowledge the absence of universally defined standards for data requirements in maintaining a successful AI model. Consequently, engineers must remain mindful of the dynamic nature of data-centric AI, with specific needs varying across industries and applications to achieve desired outcomes.
To ensure high accuracy, a multi-faceted data optimization approach is required. Engineers implementing data-centric AI are using various industry best practices, such as reduced order modeling, data synchronization, digital distortion, and image augmentation, to achieve optimal accuracy. Engineers are increasingly adopting reduced order modeling to prioritize the data fed into a model, enabling faster processing and reduced computational requirements. While maintaining data quality, this approach may involve a slight compromise in fidelity. In image-based applications like object detection or classification, engineers address potential gaps in training data by generating additional copies through re-capturing or augmenting the original images. This ensures an abundant volume of data for effective model training. Data synchronization plays a crucial role in aligning the data used with the specific requirements of the application. For instance, if an AI model needs to make hourly predictions, it should be trained using corresponding hourly data inputs to optimize its performance.
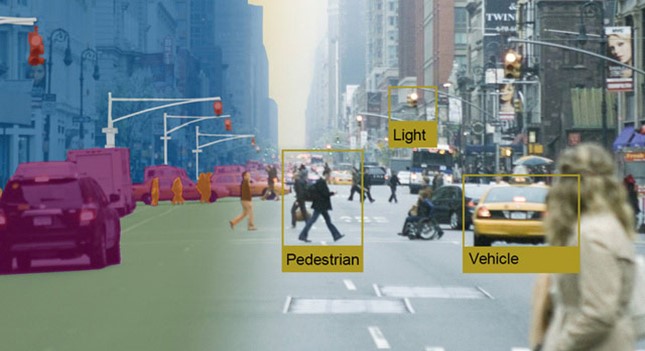
As data quality improves, so too will engineers’ ability to tackle bias. Improved data makes it easier to recognize bias, providing engineers with the insights needed to ensure adequate data collection to provide a representative outcome in vital fields like healthcare.
Emerging Frontiers of Innovation
The increased emphasis on data and its positive impact on model outcomes has led to the application of dynamic data-centric AI in various niche areas across industries. Wireless technology is one such example. Data optimization techniques have revolutionized the design of digital predistortion filters, which proactively modify signals to achieve a satisfactory noise level amidst competing signals. In the field of LiDAR, data-centric AI plays a role in evaluating and cleansing error-prone data provided by sensors. By bringing sensors closer to their intended functions and performance levels, engineers can rectify live operational data that is crucial but inaccurate.
In healthcare applications, the integration of image and signal data allows engineers to fine-tune 3D imaging machines for more precise tumor analysis, lung health measurement, and potential applications in COVID-19 screening.
Automotive engineering also benefits from data-centric AI by enabling a more comprehensive understanding of battery sensor data, including voltage and average temperature. This enhances the accuracy of state-of-charge estimation, acritical aspect in the design and enhancement of electric vehicle batteries.
There are a number of experiment-based and data preparation tools that can assist engineers in implementing data-centricity into AI models. Data-centric AI brings code and code modification to the upfront of the design process, as model code remains mostly constant. MathWorks’ Experiment Manager application has the ability to test coding protocols aimed at data optimization, allowing engineers to evaluate potential AI modeling improvements through data quality adjustments. Engineers also find value in data preparation apps that enable quick and automated data labeling, along with pre-processing libraries often used in applications relying on signal data.
Empowering the Era of Data-Centricity
As research progresses in the field of data-centric AI, the importance of collaboration among multidisciplinary teams becomes evident. While focusing on efficient modeling techniques, engineers must recognize the essential role played by data scientists inleading modeling efforts and the engineers responsible for providing the necessary data. Close collaboration between these two groups is crucial to ensure effective model development. Data-centric AI facilitates this collaboration by demonstrating how data enrichment can support the creation of models that engineers may not have considered initially.
The adoption of data-centric AI by engineers in various industries is rapidly increasing, leading to enhanced data quality and improved model accuracy. Beyond the advancements in accuracy across a wide range of applications, data-centric AI has the potential to generate a significant societal impact through its widespread use and the promotion of collaboration among different stakeholders.
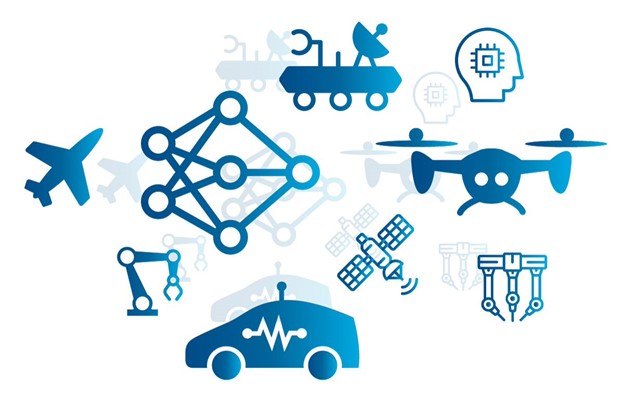